Beat the Bias – Predictive B2B Sales Modeling (Part 1)
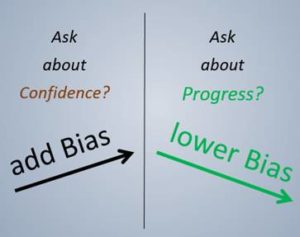
What is “Predictive Analytics”? According to SAS (the software company), it is “the use of data, statistical algorithms and machine learning to identify the likelihood of future outcomes based on historical data.”
Since “the use of data” opens this definition, it raises the question “What data do we use?” I have seen sales funnel forecasting cause serious damage, since line item data has many assumptions buried in it. These assumptions disappear in the consolidated data used by executives to run the business. To be blunt, the forward-looking data provided by B2B sales teams has a natural bias embedded in it. This is not just random “Garbage in /Garbage out”. The bias is typically “too optimistic”. The bias reasons can be assessed, and understood in a predictable way. Then a bias removal plan can be designed.
The bias removal plan will typically involve a new model of how corporate buyers actually make decisions to buy or refuse a big-ticket purchase. In this series of articles a new modeling approach will be described. But first, let’s review some reasons for forecasting bias.
As context, the key data used in B2B Forecasting has 4 common components – Deal $ value, Deal % Probability, Date of closing, and Date of revenue. All of these can be distorted by people involved in creating the data. Here are some of the red flags:
- Managers pressed for time, asking “Have we submitted a proposal?”
- Managers seeking to quantify % Probability by asking for “Confidence level” of sales person
- Sales people steadily increasing % Probability every month, based on activity
- Ignoring competition in assessing % Probability (and “do nothing” is one of the competitors)
- Using optimistic dates – for both closing and revenue
The Origins of Bias
Let’s dig deeper into a key question … what are the sources of bias? In my experience here are some examples:
- Customers ask for a proposal too early; we prepare one, we send one – then a stalled deal emerges
- We rely on generic sales tools (products, applications, general ROI claims… cool presentations and videos)
- Sales process does not build support for customer-centric specific decision drivers – e.g. Business needs, Budget ROI case, Time context, Internal Proposal
Also, there are some common business behaviors which have a negative impact on forecast accuracy. Here are some examples.
Bad Habits of Sales Executives:
- Ask sales team if a “proposal” has been submitted (with subtle pressure for more proposals)
- Ask sales people for their “confidence level”
- Push sales people for higher confidence levels
- Push for larger forecasts, in an effort to get larger revenues
Bad Habits of Sales people:
- Offer an early proposal to a prospect
- Submit a proposal quickly, then chase it
- Perceive a management question about “Confidence” as a personal evaluation
- Think about “how we can win” without consideration of “how we could lose”
- Overlook the importance of the B2B buyer’s “Internal Proposal”
Could the underlying model be truly “Predictive”?
So some danger could arise using “Predictive Analytics” by itself, since it might just be a way to compensate for underlying bias. The analytics would work better if the basic model for sales were to shed some of its bias. This can be done, and bias-prone data gathering can be replaced by estimating rules built into the sales process. These estimating rules are part of a new buyer-centric “Predictive Modeling” process that you can use for any B2B sales team. The process may have errors, however the errors tend to be random, and thus cancel each other out. More on the principles of Predictive Modeling design will follow. (Hint: Design will be based on modeling Progress, built into a sales staging strategy.)
Follow Us
Follow us on LinkedIn for updates.